Data Center
Data Center
The Ultimate Guide To Your AI-Ready Data Center
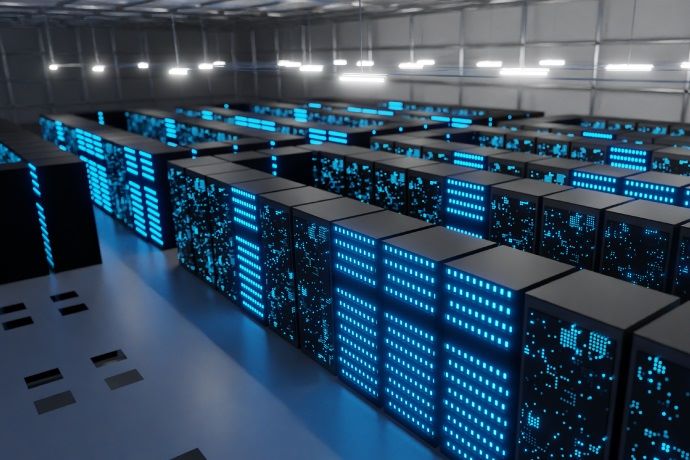
As artificial intelligence (AI) continues to advance, the importance of data centers has become increasingly pivotal. The demand for robust, scalable, and efficient data centers is surging. To meet these demands, data centers must be designed and optimised for AI workloads, ensuring they can support the high computational and storage needs of modern AI applications.
AI workloads differ significantly from traditional IT tasks. They involve massive data processing, high-performance computing, and complex algorithms that require substantial computational power. Data centers designed for AI must handle parallel processing, high throughput, and low-latency communications. Understanding the nature of AI workloads—whether they are training deep learning models, running real-time analytics, or performing large-scale simulations—is the first step in designing an AI-ready infrastructure.
High-Performance Computing (HPC) Infrastructure
At the heart of an AI-ready data center is high-performance computing infrastructure. AI applications, particularly deep learning models, require powerful GPUs and specialized hardware accelerators. These components are essential for processing large datasets and training complex models efficiently. A modern data center should incorporate state-of-the-art GPU clusters, Tensor Processing Units (TPUs), and other accelerators designed to meet the demands of AI tasks.
Scalable and Flexible Architecture
Scalability is a key factor in any AI-ready data center. As AI applications grow and evolve, so too must the data center infrastructure. Implementing a scalable architecture allows for the addition of new resources such as additional servers, storage, or networking capabilities—without significant downtime or reconfiguration. Modular data center designs, which support rapid scaling and flexible expansion, are particularly well-suited to accommodate the dynamic nature of AI workloads.
Advanced Cooling Solutions
AI and HPC systems generate substantial heat, necessitating advanced cooling solutions to maintain optimal operating conditions. Traditional cooling methods may not suffice for the high-density deployments typical of AI environments. Innovative cooling solutions, such as Rear door Heat Exchangers, In-row cooling, Direct liquid to chip cooling and immersion cooling, can efficiently manage the heat output of densely packed hardware. Proper cooling is critical not only for performance but also for prolonging the lifespan of sensitive electronic components.
Robust Network Infrastructure
AI applications often require high-speed data transfers and low-latency network connections. A robust network infrastructure is essential to support these needs. This includes high-bandwidth network interfaces, low-latency switches, and efficient data routing. Data centers must be equipped with redundant network paths to ensure uninterrupted connectivity and to handle peak loads efficiently.
Enhanced Data Security
Data security is a paramount concern in AI environments. With the increasing volume of sensitive data being processed, protecting against unauthorized access and cyber threats is crucial. Implementing comprehensive security measures, such as encryption, access controls, and intrusion detection systems, helps safeguard data integrity and confidentiality. Regular security audits and compliance with industry standards further enhance the security posture of the data center.
Energy Efficiency and Sustainability
As AI workloads can be resource-intensive, energy efficiency and sustainability are vital considerations. Data centers should prioritize energy-efficient components and practices to reduce operational costs and environmental impact. Employing green energy sources, optimising power usage effectiveness (PUE), and implementing energy-saving technologies contribute to a more sustainable and eco-friendly data center.
Effective Data Management
AI applications generate vast amounts of data that must be stored, managed, and analyzed efficiently. Implementing effective data management practices, such as tiered storage solutions and data lifecycle management, ensures that data is readily available when needed and stored cost-effectively. Colocation data centers support high-speed storage solutions and scalable storage architectures to accommodate the growing data requirements of AI applications.
Automated Management and Monitoring
Automation plays a significant role in managing AI-ready data centers. Automated management systems can streamline operations, optimize resource allocation, and quickly identify and address issues. Monitoring tools that provide real-time insights into system performance, resource usage, and environmental conditions help maintain the health of the infrastructure and support proactive management.
Conclusion
Designing and managing an AI-ready data center involves careful consideration of various factors, including infrastructure, scalability, cooling, networking, security, energy efficiency, data management, and automation. Yotta’s hyperscale data centers exemplify these principles, offering state-of-the-art infrastructure, robust connectivity, and advanced cooling systems to provide the ideal foundation for AI-powered applications. Yotta’s data centers in India are designed to support the most demanding AI applications, ensuring reliability, efficiency, and security. As AI continues to transform industries, Yotta remains at the forefront, offering the infrastructure necessary for success in a rapidly evolving digital landscape.